To effectively discuss the topic of Artificial Intelligence AI in Clinical Trials Planning and Design, we need to discus what is meant by Artificial Intelligence in this context. If one looks up definition of Artificial Intelligence (AI), one finds many different varieties. This is not surprising as we all have seen many different applications of technologies designated as AI for a specific purpose. As the definition is concerned, they all revolve around the idea that it is defined as the ability of a computerized system to perform tasks commonly associated with intelligent beings. As intelligent beings, humans are capable of understanding complex relationships and draw learning from their interactions with them. Likewise, it is expected that an ‘intelligent’ machine would be capable to form and understand complex relationships between entities and draw new learning and insight from them, provided they are ‘thought’ the basic rules of Processing>Organizing>Analyzing>Learning paradigm of information at hand.
However, since our passion is driven by the need to optimize the Clinical Research in general and Clinical Trials in particular, we have naturally, been occupied by looking into the use of AI in Clinical Trials Planning and Design. One may ask, why this early area of the Clinical Trial life-cycle? But the answer is quite simple – it is because this early phase of the Clinical Trial life-cycle is the one where production of unstructured data seems to be the norm. We all seem to be accepting of the fact that Clinical Trials are planned, designed and documented in an unstructured form of Clinical Development Project documentation and Clinical Protocol documents – all typically defined and provided in MS Word. Even more so, we all seem to be content on waiting for such documents to be fully written, reviewed and approved before anything is conducted to actually execute the trial.
Does it really have to be that way? Is there a better way of doing it and how could we benefit from the AI in the process?
We believe that there is a better way and hope to take you through the journey of Structured Study Definition (SSD) combined with the Protocol Quality Gates* and how it could unleash the power of AI in this early phase of the Clinical Trial life, namely Planning and Design.
Artificial Intelligence AI in Clinical Trials: Technology
From technology perspective, the AI paradigm within the clinical trial planning and design can be implemented using the existing technology to process the information and make it readily available for any prediction and evaluations on the appropriateness of the trial design, given the trial design specifics and past experiences (see Figure 1).
For text processing and mining from unstructured sources one could apply Natural Language Processing (NLP) techniques such as segmentation, tokenization, pattern matching, categorization and entity recognition. Depending on the NLP capabilities one can deduce the underlining structure in seemingly unstructured texts.
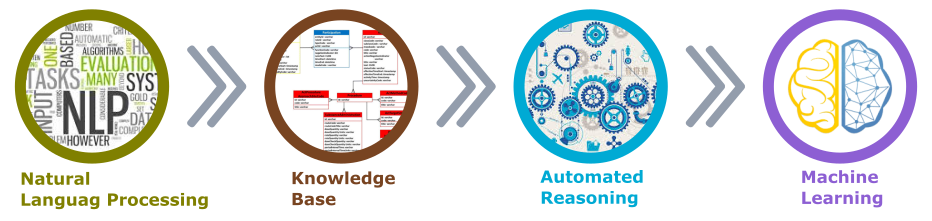
Figure 1. Enable Artificial Intelligence
Knowledge Base is where the extracted and processed unstructured source information is organized and enriched to enable the automated learning algorithm and processing of the data to structure it for the Machine Learning. The Machine Learning area forms the core of the AI application to enable the evaluation and predictive information of the Clinical trial, based on the design characteristics and past trials experience.
Today, the majority of the research organizations are still employing the document-centric approach to Clinical Development and still would be exposed to a wealth of unstructured information which cannot easily be transformed into a knowledge base. Thus, remaining outside of the realm and transforming power of the AI technologies.
Artificial Intelligence AI in Clinical Trials: Data Processing
Looking at the Artificial Intelligence in Clinical Trials from the data processing perspective, we recognize the importance of the Structured Data. Only, the data structured in the appropriate data model or flexible forms , based on which data models could be derived, can be efficiently utilized by the automation algorithms to prepare it for the prediction modeling (see Figure 2).
As we all agree that Machine Learning algorithms learn from data, in order to make that learning meaningful, the data that is being fed to such algorithms must be the right data for the problem that is being addressed.
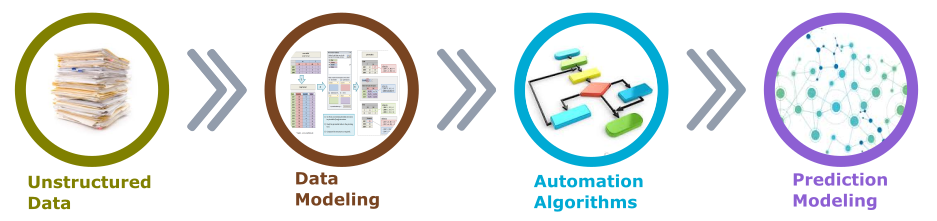
Figure 2. Structured data supports predictive modeling
Without the transformation from unstructured to structured data models, any effective data automation and machine learning remains elusive.
Artificial Intelligence AI in Clinical Trials: Business Value
Business-wise, two major drivers behind the application of AI are:
- Automate manual work
- Uncover hidden insights
How would these drives be applied in Clinical Trials Planning and Design area?
When planning and designing a new clinical trial, we typically look for reference trials for that indication and/or mechanism of action, within the industry or our internal trails from earlier projects. Where do we find them? – in publications, trial registries and document management systems. The whole process would be a lot more effective if all that information is available at one’s fingertip either by searching, drill-down even in a ‘Siri-like’ or ‘Google Assistant’ fashion:
“Looks like you are working on a clinical trail in indication … would you like to see other similar trials ?”.
All of that would be possible, once the protocol information and study reports are stored within a knowledge base in the structured form (Figure 3).
Predictive insights would be available based on the study design characters and study population combined with the experiences from previous similar trials in the past projects.
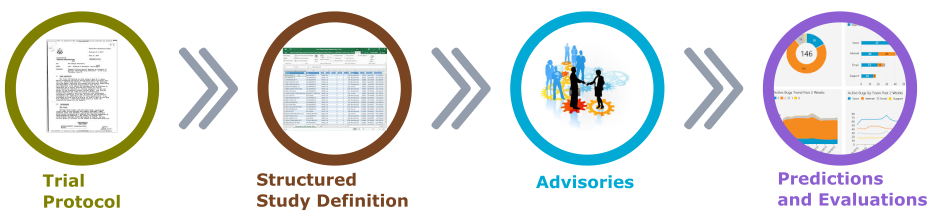
Figure 3. Artificial Intelligence in Clinical Trials Planning and Design
To ensure successful introduction and adoption of the AI in the Clinical Development space, in general, requires a certain effort in the Cultural Shift Management. That necessitates constant attention on the business values enabled and outcomes derived based on the AI technology, keeping your teams excited about AI application, training resources to benefit from the new pool of data available and in information signaling as well as data analytics, and last but not least capability to explore cross-channel evaluations and insight derivation.
In any case, AI will evolve gradually, like any other technology, with more capabilities being developed and available every day, for those capable to derive benefits from it. However, the challenges in the Clinical Development, in general, remain and would need to be addressed for any successful implementation of AI technology and concepts:
- Silos (data is in silos across Clinical Development value chain)
- Expertise gap (Data information scientists are scarce and expensive, which requires appropriate trainings of own resources)
- Infrastructure (Cost prohibitive due to powerful computing requirements, which may be addressed by IT in the cloud strategies)
- Mindset (‘AI is science fiction and out of reach’, which can be addressed by gradual introduction and value demonstration)
Conclusion
Artificial Intelligence in Clinical Trials Planning and Design has a potential to drastically change the way clinical trails are defined. The major benefits would provide the capability to apply the knowledge and learning from the previous trials within the Clinical Project or within the same indication. Additionally, the structuring of the Clinical Protocol information would enable derivation of new insights by applying AI analytics and timely (just-in-time) provision of qualified information (based on Protocol Quality Gates) to other downstream processes such as Clinical Supply, Trial Feasibility, Trial Setup, etc.
However, the major obstacle in the way seems to be the traditional document-centric approach to Clinical Trial Planning and Design. To overcome such obstacle, intilaris is introducing the SSD (Structured Study Definition) in the Clinical Trials Planning and Design process, and by doing that provides the structured data Knowledge Base which forms the basis for application of AI technologies.
Please reach out to us for an independent and expert consultation on this topic.